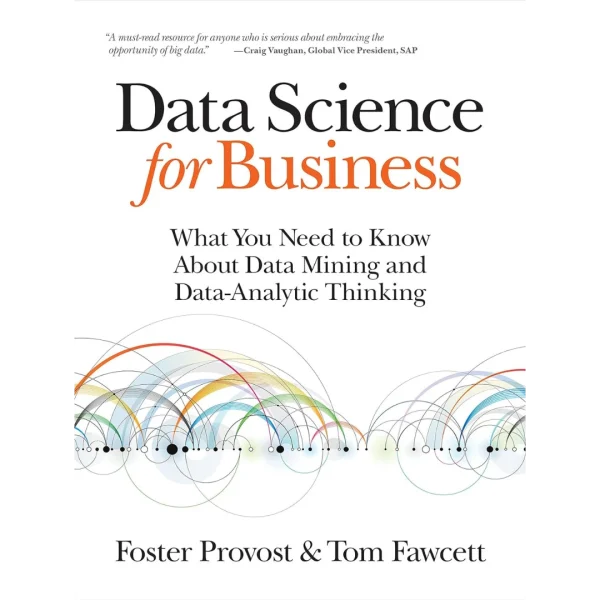
Data Science for Business: What You Need to Know about Data Mining and Data-Analytic Thinking
Original price was: $49.99.$11.00Current price is: $11.00.
PDF 2,81 MB • Pages: 382
- 100% Satisfaction Guaranteed!
- Immediate Digital Delivery
- Download Risk-Free
Written by renowned data science experts Foster Provost and Tom Fawcett, Data Science for Business introduces the fundamental principles of data science, and walks you through the “data-analytic thinking” necessary for extracting useful knowledge and business value from the data you collect. This guide also helps you understand the many data-mining techniques in use today.
Based on an MBA course Provost has taught at New York University over the past ten years, Data Science for Business provides examples of real-world business problems to illustrate these principles. You’ll not only learn how to improve communication between business stakeholders and data scientists, but also how participate intelligently in your company’s data science projects. You’ll also discover how to think data-analytically, and fully appreciate how data science methods can support business decision-making.
- Understand how data science fits in your organization―and how you can use it for competitive advantage
- Treat data as a business asset that requires careful investment if you’re to gain real value
- Approach business problems data-analytically, using the data-mining process to gather good data in the most appropriate way
- Learn general concepts for actually extracting knowledge from data
- Apply data science principles when interviewing data science job candidates
Reviews
There are no reviews yet.