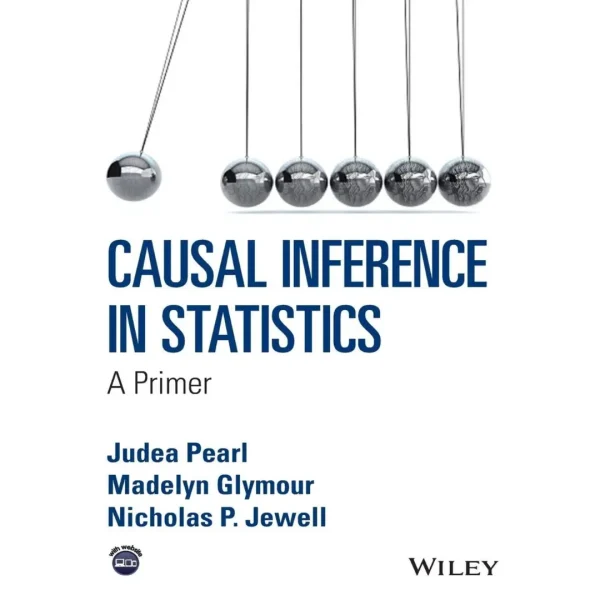
Causal Inference in Statistics – A Primer
Original price was: $50.95.$19.99Current price is: $19.99.
PDF 1,50 MB • Pages: 159
- 100% Satisfaction Guaranteed!
- Immediate Digital Delivery
- Download Risk-Free
You must be logged in to post a review.
$50.95 Original price was: $50.95.$19.99Current price is: $19.99.
PDF 1,50 MB • Pages: 159
Many of the concepts and terminology surrounding modern causal inference can be quite intimidating to the novice. Judea Pearl presents a book ideal for beginners in statistics, providing a comprehensive introduction to the field of causality. Examples from classical statistics are presented throughout to demonstrate the need for causality in resolving decision-making dilemmas posed by data. Causal methods are also compared to traditional statistical methods, whilst questions are provided at the end of each section to aid student learning.
You must be logged in to post a review.
Reviews
There are no reviews yet.